Dave: Today, we’re diving into the integration of AI into business workflows. We’ll explore whether the initial hype is settling, how we are guiding our own clients through common challenges, and the typical needs businesses face in this evolving landscape.
When Chat GPT launched a couple of years ago, it sparked a frenzy around generative AI. Some were claiming it marked the beginning of a new industrial revolution, set to transform the world as we know it. Let's discuss whether those predictions are coming to fruition.
Justin: I think the initial excitement stemmed from the surprise at how advanced GPT-3.0 and 3.5 were, especially compared to what people expected based on their experiences with relatively static chatbots. Also, AI researchers were surprised at the capabilities of the transformer approach - so it started out as a hype well deserved. The reality of harnessing that, and building it into new business models and new applications, has paid off in some areas, while in others people are still rapidly experimenting but haven't yet found a business model that works. The big language model providers are losing money on this currently, but they’re betting on the future. The next generation of models, and the generation after that, will be the engine of automation and applications like driverless cars and taxis, advanced robotics, medical and climate research, synthetic biology and more.
I’ve heard the hype curve described only this week as AI-washing. Some businesses and consultants seem to be capitalising on the fear of missing out by producing generic content about AI's potential and limitations. I think we’ll go through a cycle over the coming months until the next real upshift in capabilities happens and, off the back of that, more concrete use cases in the real world are validated and used by people.
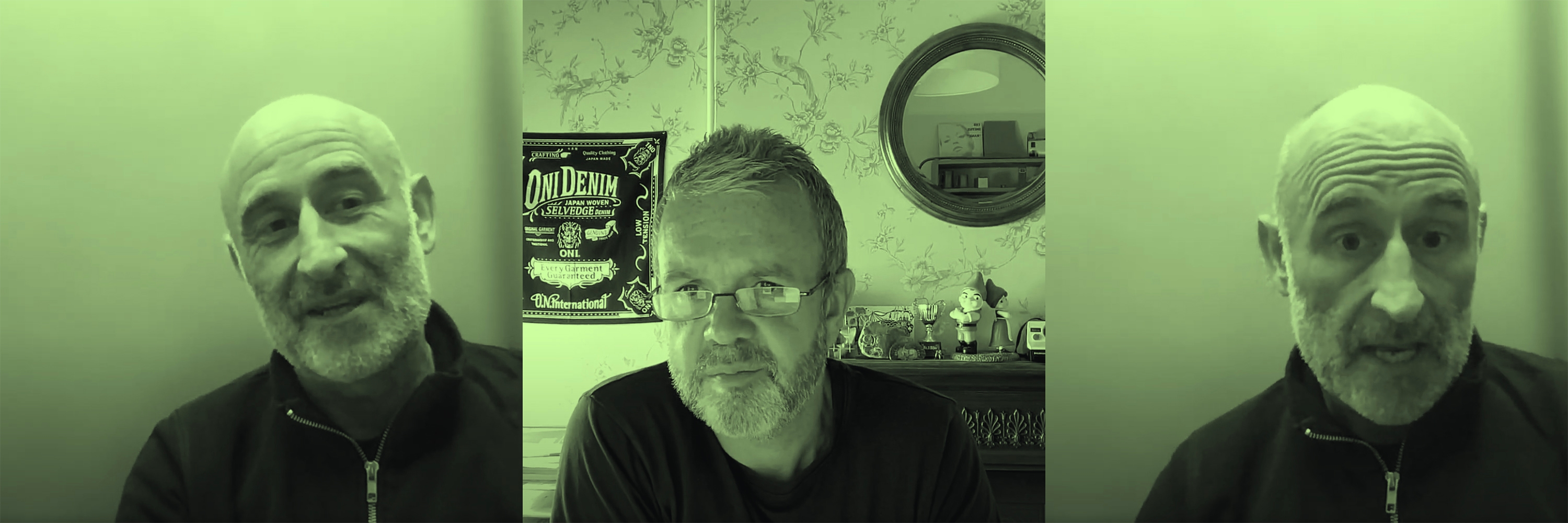
Dave: What I've been seeing over recent weeks feels like AI’s being talked up more for its potential to support humans, rather than replace us…
Justin: Yes, Juan Ferres’ book AI for Good: Applications in Sustainability, Humanitarian Action, and Health came out last week, which talks through use cases of AI being used for good, and where it can do so in future. But, certainly in areas such as scientific research and healthcare for example, there are lots of potentially great applications.
Dave: AI for good feels very current. Until recently, it felt like we were going to have to go through really difficult times before things could improve. Bill Gates said that the energy being hammered by AI now would be offset because it would soon start saving a lot of energy. But that needs to happen now, right? Our energy consumption isn't going to decrease…
Justin: Well no, we're not going to reduce our energy consumption any time soon. New technologies and growing populations are going to keep increasing demand. Currently AI is very energy-inefficient, but newer models are becoming more efficient, using fewer compute cycles for training and delivering results. But AI is still expensive to run, and there’s limited cost-benefit analysis available. People are betting on AI's future potential to improve efficiency in research, engineering and manufacturing, but for now companies are building bigger data centres, which consume even more energy to support AI advancements.
It’s interesting. The trajectory looks like those problems may be solved quicker than first thought, so actually having a high upfront cost in terms of carbon footprint now should play out sooner than the 2050 catastrophic climate heating that's being predicted. It's a race though, and we don't know for sure.
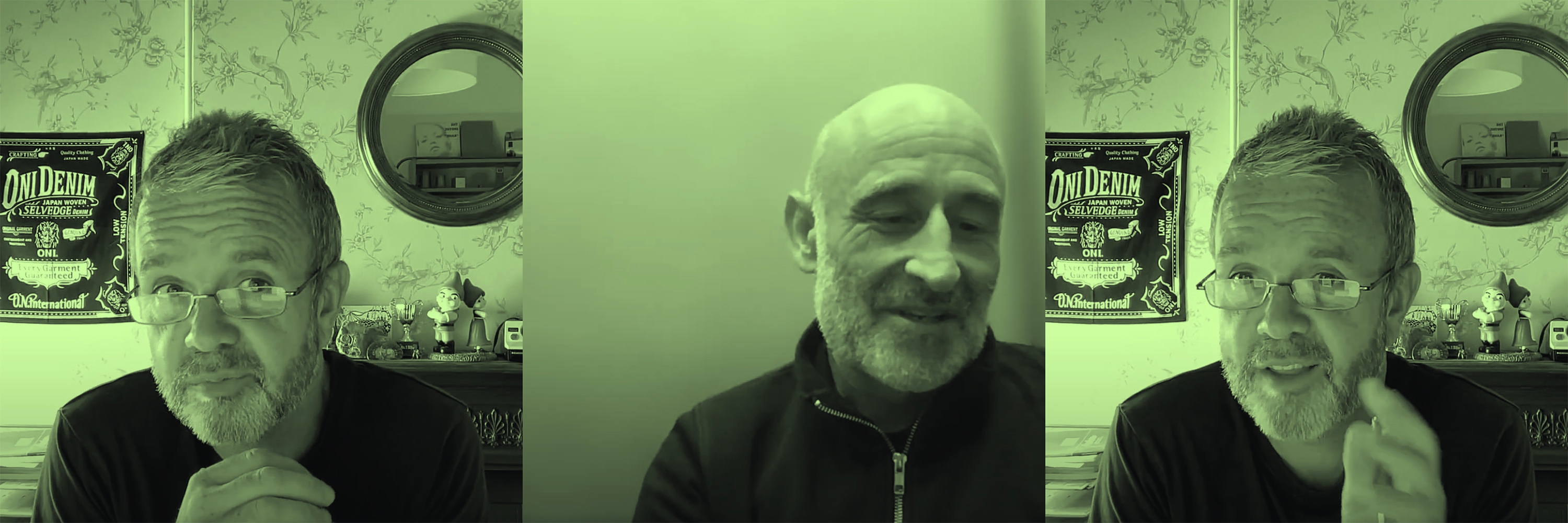
Dave: Only a year ago we had the big boys like Goldman Sachs saying hundreds of millions of jobs are going to be lost, or at least become massively downgraded in skills. It feels now like this was over-egged, wouldn’t you say?
Justin: All innovation causes changes in the job market, and the skills people need to learn in order to be able to find employment; AI is no different. You still need a reasonable level of design know-how in order to use a generative AI model to create good design work, because you need to recognise what goes into good design in the first place, and use the right language etc.
Dave: And the human vision and understanding that nothing short of a human would be able to originate…
Justin: Yeah, or at least to be able to curate that and select it. There's already a kind of sub-movement betting on the idea that maybe some people will want human-only design and interactions. I can easily envisage a whole artisan movement establishing itself off the back of it which will actually probably create jobs in that area. We adapt, and despite how much everybody moans about going to work, we invented work as a species and…
Dave: Ants invented work, mate…
Justin: Education needs to catch up, and people need to learn new skills and new ways of working alongside AI being the emerging technology.
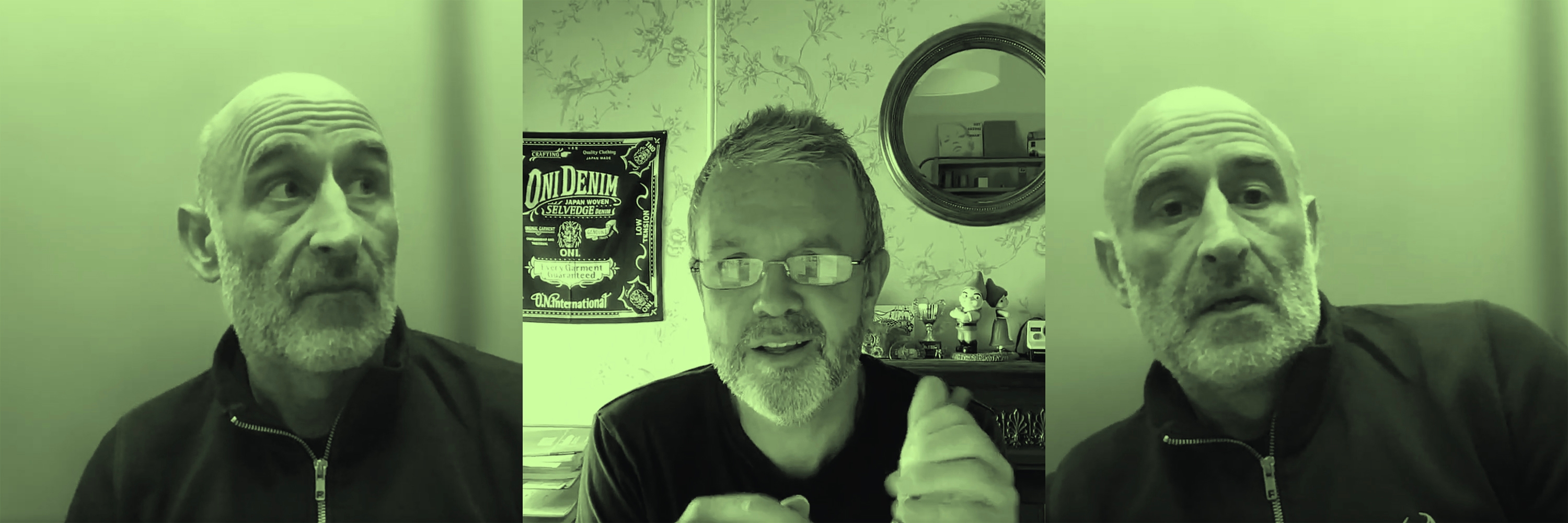
Dave: So on that note, talk to me about a specific example of AI integration we've worked on for clients' platforms that has supported or shaped specific human roles, or their way of working.
Justin: I think there are two areas. There's the automating away of quite repetitive, relatively dull tasks where, although there's value in the output, there’s not much value through the input stage. Automating things like classification of content and documents for example, and data is an example of that.
Dave: Do you have an example of a typical client needing that kind of work?
Justin: Any content owner, publisher, insights professional or large enterprise with vast amounts of content that employees need easy access to spends significant time and money tagging that content. But existing search tools often fall short in finding content, especially when it comes to understanding intent from a natural language query. Then there's the AI coworker aspect that we've been working on, allowing people to find content or interrogate data again in a much more human way, so they can spend their time focused on understanding the insights in that data, and not just trying to write the best SQL query to get that data out in the first place.
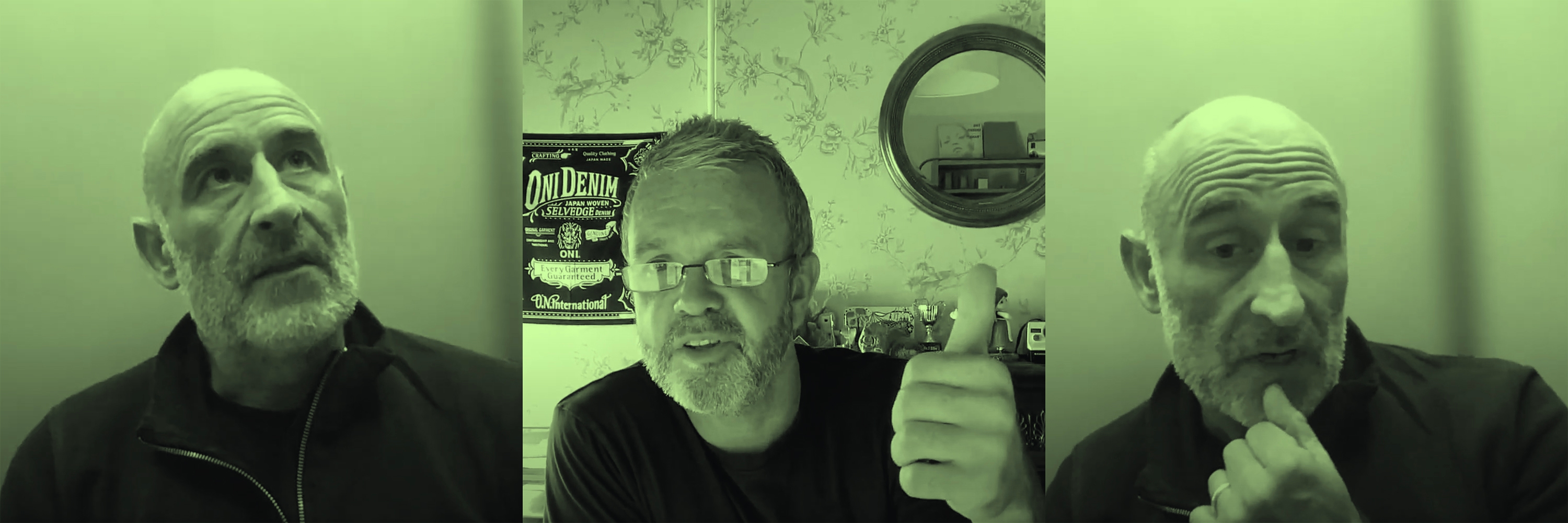
Dave: They need to understand the value of everything, and AI is no different. So as well as the operational areas, how have we been helping our clients to assess and prioritise AI against their strategic objectives?
Justin: There are the operational areas as you say, where you can have quite a high impact with relatively low cost investment to automate away repetitive tasks, and the ones that may be more error-prone. Therefore you're not just gaining value in efficiency, but also in the quality of the actual output. Classification and organisation of documents, or moving and transforming data or tasks - when done manually - inevitably end up with human errors. Automating processes governed by an agentic flow can be set up to be very accurate and clean.
Going on from that, as the capabilities of the generative models have expanded, and those capabilities are being integrated into people's design, coding and data analysis tools for example, you can have a very positive impact by upskilling your existing teams to use and get the most out of those capabilities by understanding how the models work, how you prompt them, and how you then refine your prompts when you’re prompting over and over to get the right result.
This all hinges off the idea of impacts versus cost analysis. How much impact are you making in time or cost savings? Or actually is the value-add around someone being more creative or strategic because of those time savings.
Customer experience is another key area, where businesses can use already proven AI implementations to build value for customers. This can be AI-enabled micro interactions within the customer journey, or it can be larger scale improvements such as replacing more complex user journeys with agentic flows.
Another area currently being busily experimented with is the question of what current AI capabilities, and immediate future AI capabilities, mean for our product development. What can we be building - what can we introduce to our product and service suites that we don't currently have - but should have? That's much more about understanding their business models, the data and content they own, and then working with them to very quickly produce proof-of-concepts or prototypes for ideas. For a current scientific publisher client for example, prototyping has shown them they can monetise content they couldn't previously, so the next phase is to roll that out to all of their portals.
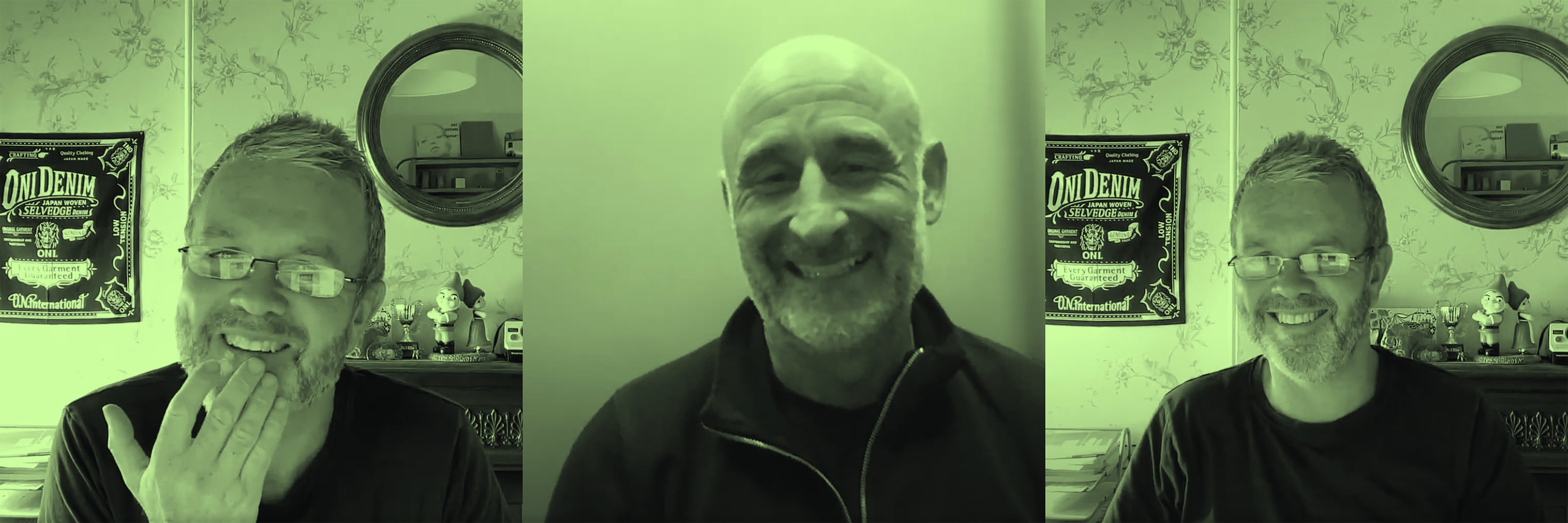
In summary, there are three key areas to focus on:
- Achieving quick wins and upskilling the business and its users to be AI-ready.
- Enhancing customer experience.
- Assisting in reviewing product and service roadmaps to identify where AI capabilities can be integrated.
Dave: And to aid this, are we seeing our own clients increase their focus on training their teams in AI literacy and educating them on how it works, including its potential applications and ethical considerations?
Justin: Yes absolutely. They are, and they're giving their teams time to experiment with things like CoPilot and the various other tools out there. The savvy ones are embracing AI with a proactive mindset, and weaving it into their company culture. They’re turning AI from a perceived threat into an exciting opportunity to work more efficiently and deliver greater value. It's absolutely crucial for businesses to understand and adopt this approach.